Title: Multilingual Image Corpus
Duration: 2021
Funding: The Multilingual Image Corpus (MIC 21) project was supported by the European Language Grid project through its open call for pilot projects. The European Language Grid project has received fund- ing from the European Union’s Horizon 2020 Re- search and Innovation programme under Grant Agreement no. 825627 (ELG).
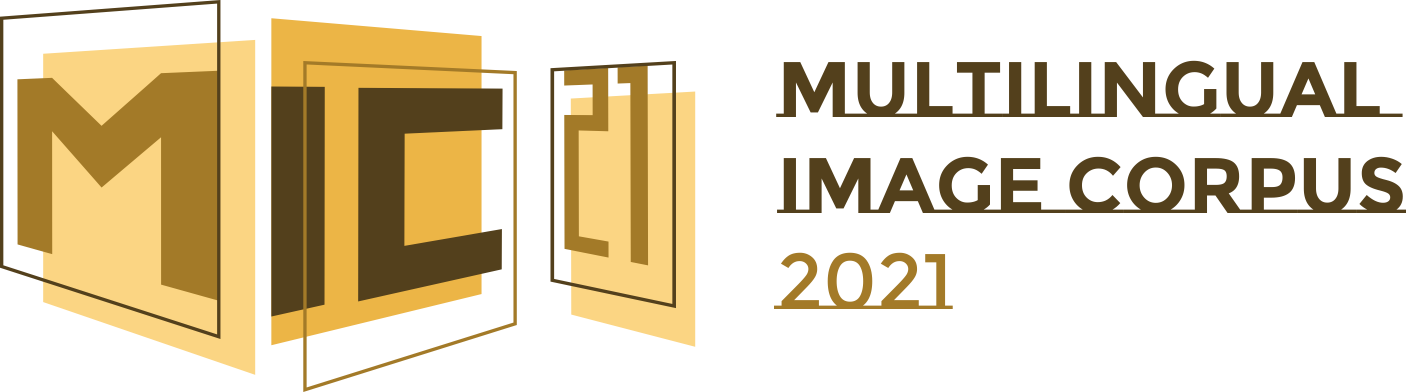
Team members
Principal Investigator: prof. Svetla Koeva
Team members: prof. Svetla Koeva, Ivelina Stoyanova, Yordan Kralev, assist. prof. Svetlozara Leseva, assist. prof. Valentina Stefanova, assist. prof. Tsvetana Dimitrova, assist. prof. Maria Todorova, Hristina Kukova, Victoria Petrova, Kristiyan Lyubenov, Krsatyo Gigov.
Summary:
The Multilingual Image Corpus consists of an Ontology of visual objects (based on WordNet) and a collection of thematically related images whose objects are annotated with segmentation masks and labels describing the ontology classes. The dataset is designed both for image classification and object detection and for semantic segmentation. The main contributions of our work are: a) the provision of large collection of high-quality copyright free images; b) the formulation of the Ontology of visual objects based on WordNet noun hierarchies; c) the precise manual correction of automatic object segmentation within the images and the annotation of object classes; and d) the association of objects and images with extended multilingual descriptions based on WordNet inner- and interlingual relations.
The shift of traditional data fusion methods challenged by multimodal big data motivates the creation of a new image corpus, the Multilingual Image Corpus, which is characterised by carefully selected images that illustrate thematically related domains and precise manual annotation for segmentation and classification of objects in the images.
Our main goal was to develop an image dataset distinguished with the following main features: (a) a large image collection containing thousands of images and annotations; b) annotation classes belonging to a specially designed Ontology of visual objects; c) automatic object segmentation and classification evaluated by experts; d) the object classes and attaching definitions of concepts translated in at least 20 languages. An important goal with great impact for the development of different applications is the open distribution of the dataset.
To accelerate the manual annotation, we envisaged the development of an image processing pipeline for object detection and object segmentation using pre-trained models. We planned also to deliver a reliable service for automatic annotation of objects in images: using containerisation technologies; training and re-training of models; connecting the existing tools and machine learning frameworks. During the dissemination we aimed to engage the professional communities to discuss different solutions and best practices and to demonstrate and promote the ELG and MIC21 project.
Overall, the project aims are directed towards results which can be directly implemented in: automatic identification and annotation of objects in images (a prerequisite for effective search of images and (within) video content), automatic annotation of images with short descriptions in European languages.
We have divided the annotation process into four main stages: a) definition of an Ontology of visual objects; b) collection of appropriate images; c) automatic object segmentation and classification; and d) manual correction of object segmentation and manual classification of objects. The dataset contains 4 thematic domains (Sport, Transport, Arts, and Security), which group highly related dominant classes such as Tennis player, Soccer player, Limousine, Taxi, Singer, Violinist, Fire engine, and Police boat in 130 subsets of images. We have used the COCO Annotator, which allows for simultaneous working within a project.
Domains | Number of subsets | Number of images | Number of annotations |
SPORT | 40 | 6,915 | 65,482 |
TRANSPORT | 50 | 7,710 | 78,172 |
ARTS | 25 | 3,854 | 24,217 |
SECURITY | 15 | 2,837 | 35,916 |
TOTAL | 130 | 21,316 | 203,787 |
The Multilingual Image Corpus provides fully annotated objects within images with segmentation masks, classified according to an Ontology of Visual Objects, thus offering data to train models specialised in object detection, segmentation and classification. The Ontology contains classes (706 representing visual objects and 147 representing their hypernyms), relations and axioms. Classes correspond (but are not limited) to WordNet concepts which can be represented by visual objects. Part of the relations and their properties are also inherited from WordNet. Axioms serve to model statements that are always true. The Ontology of visual objects allows easy integration of annotated images in different datasets as well as learning the associations between objects in images.
The images in the dataset are collected from a range of repositories offering API: Wikimedia; Pexels; Flickr; Pixabay. Creative Commons Search API is also used. Each image is equipped with a metadata description in JSON format (sample). The metadata include the following fields: dataset, file path, MIC subdataset, MIC subdataset id, image author, author url, image original size, file name, image id, image license, image source, source url last access, source name, source url, MIC21 license, MIC21 provider, MIC21 credit, MIC21 provider contact, MIC21 project url.
To accelerate the manual annotation, an image processing pipeline for object detection and object segmentation was developed. Two software packages – YOLACT and Detectron2, and Fast R-CNN models trained on the COCO dataset were used for the generation of annotation proposals.
The Ontology classes are translated into 25 languages (English, Albanian, Bulgarian, Basque, Catalan, Croatian, Danish, Dutch, Galician, German, Greek, Finnish, French, Icelandic, Italian, Lithuanian, Polish, Portuguese, Romanian, Russian, Serbian, Slovak, Slovene, Spanish, and Swedish). Openly available wordnets have been used from the Extended Open Multilingual WordNet. For the Ontology classes which are not inherited from WordNet the appropriate WordNet hypernyms are taken. Where WordNet translations are not available, some additional sources of translations as BabelNet are employed. The Multilingual translations of classes are presented in a separate JSON file which contains information about the language and the translation source (sample). The translations of the Ontology classes are accompanied by their synonyms, the concept definition and usage examples (if available in the sources). The explicit association of objects and images with appropriate text fragments is relevant for multilingual image caption generation, image-to-text alignment and automatic question answering for images and video.
We developed a Framework for evaluation and running of MIC21 models based on FiftyOne, Yolact, Detectron2 and implemented it over Mask R-CNN on Python3, Keras and TensorFlow. We pre-trained Fast R-CNN models using the Detectron2 framework with ground truth annotations which resulted in 130 models (sample) which generate bounding boxes and segmentation masks for each instance of a particular object within an image. The framework maintains an API functionality for processing new images with any of the three models: Yolact, Detectron2 and MIC21. The Framework for evaluation and running of MIC21 models is available under the Creative Commons Attribution-ShareAlike 4.0 International (CC BY-SA 4.0) license at the ELG platform. The demonstration of MIC21 dataset and the MIC21 Framework for evaluation and running of MIC21 models is available here.
The MIC21 models and model images are stored at the MIC21 repository.
The dataset (images, resized images, image metadata, annotations, Ontology classes and Multilingual descriptions of classes) is available under the Creative Commons Attribution-ShareAlike 4.0 International (CC BY-SA 4.0) license at the ELG platform and also in the MIC21 repository.
The First ELG Dissemination Event in Bulgaria was held on 11.02.2022 and attended by 121 people from academia (15), universities (48), representatives of EU or EC institutions (5), Bulgarian governmental agencies (6), tech companies (31) and the media (6). Information about the ELG Dissemination Event was broadly disseminated through DCL and IBL’s websites, through Facebook (link) and LinkedIn (link) campaigns and extensive malinglists of interested parties. The event was streamlined through DCL’s YouTube channel (link) and the recording has 300 views. The event was covered in 2 interviews by Prof. Svetla Koeva on the Bulgarian National Radio on 10.02,2022 (link https://bnr.bg/hristobotev/post/101599524) and on 16.02.2022 (link https://bnr.bg/sofia/post/101602243).
Gallery
![]() |
![]() |
![]() |
![]() |
![]() |
![]() |
![]() |
![]() |
![]() |
Publications
Koeva, S. Multilingual Image Corpus: Annotation Protocol. Proceedings of the International Conference on Recent Advances in Natural Language Processing (RANLP 2021), Publisher: INCOMA Ltd., 2021, ISBN:978-954-452-072-4, ISSN:1313-8502; series eISSN 2603-2813, DOI:https://doi.org/10.26615/978-954-452-072-4_080, 701-707.
Koeva, S., I. Stoyanova, Y. Kralev. Multilingual Image Corpus – Towards a Multimodal and Multilingual Dataset. In: Proceedings of the Language Resources and Evaluation Conference (LREC 2022). European Language Resources Association. (pdf)
Conferences
Koeva, S. Multilingual Image Corpus, The Third Annual ELG Conference META-FORUM 2021, 15.11.2021 (pdf)
Koeva, S. Multilingual Image Corpus: Annotation Protocol. International Conference on Recent Advances in Natural Language Processing (RANLP 2021), 03.09.2021 (pdf)
Koeva, S., I. Stoyanova, Y. Kralev. Multilingual Image Corpus – Towards a Multimodal and Multilingual Dataset. Language Resources and Evaluation Conference (LREC 2022). June 2022. (video)